Woojin Jung, an assistant professor in the Rutgers School of Social Work, credits her interdisciplinary education in social welfare, public policy, and development engineering for her award-winning research. In December, she was honored with the 2020 Society for Social Work and Research Outstanding Social Work Doctoral Dissertation Award for Combating Poverty Through Aid: A Critical Analysis of Alternative Models, which she wrote at UC Berkeley to fulfill her PhD in social work and development engineering. To find out more about Jung’s poverty measurement research and her contributions to development engineering, the Blum Center conducted the following interview.
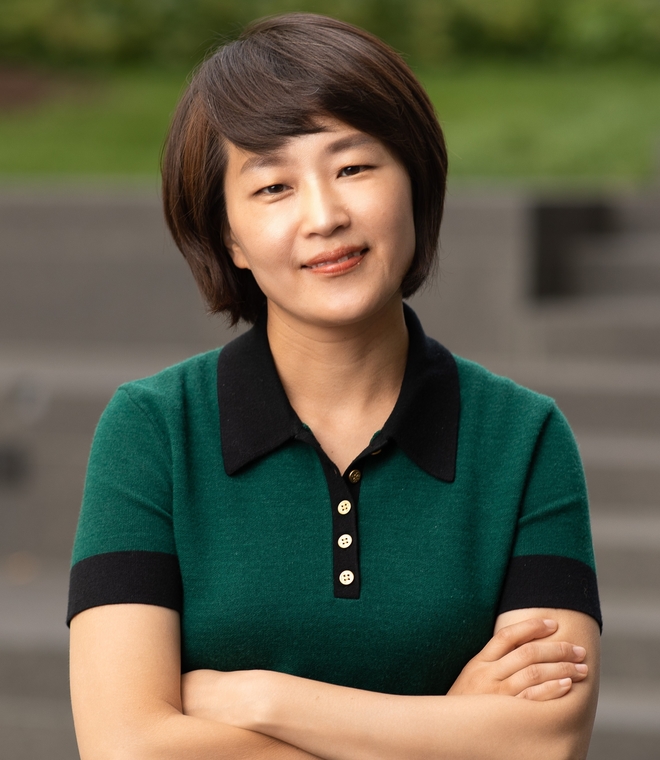
Your dissertation examines the discrepancies between different global poverty measures and brings that analysis to bear on identifying the salient dimensions of poverty in developing countries. What were your most surprising or meaningful takeaways from this analysis?
One surprising finding is that the discrepancies between the two approaches to poverty were larger than I thought. For instance, in Cambodia in 2010, only 10 percent of the population was poor by a $1.90 poverty measure, but almost half of the population was living in poverty by multidimensional measure. In development agencies, when it comes to the usage of indicators, income measures dominate but this study shows that each measure requires attention. How to incorporate multiple measures is another issue. Policymakers and research communities can juxtapose various measures one by one, taking a dashboard approach, but I want to take a systemic account of discrepancies. It was interesting to me that exceptions and mismatches between measures are not always bad but may serve as interesting sources of information and have the potential to be used as a policy instrument.
The most unexpected finding was that some evidence of the match between needs and policy intervention, which I would call the diagnosis and prescription match. My study finds that the “capability poor” countries receive marginally higher social sector aid relative to economic sector aid.[1] Social sector aid aiming to address capability poverty has skyrocketed since the beginning of the 2000s, significantly outpacing the economic and production aid. The result of the analysis tells us that higher rate of social sector aid is not uniform but more in countries where poverty is more multidimensional. Further research can expand this discussion by analyzing whether the considerable policy shift favoring the social sector was in response to the growing rate of “capability poor” countries to “income poor countries” or in response to the large magnitude of capability poverty as relative to income poverty. As for the individual country, more attention can be paid to outliers lacking the diagnosis and treatment match[2]
Given what you know about discrepancies between measures of international poverty and advances in technology to better measure poverty, how can the development community better distribute aid in, say, Myanmar, where you focus some of your paper?
I would say that development communities should be more clear and consistent about the definition and concepts of poverty and policy responses to address poverty. Rhetorically, the development community calls for tackling “poverty.” However, in terms of aid targeting, they equate the meaning of poverty with low gross national income. Strictly speaking, poverty and low gross national income inform needs from different angles. The poverty rate exclusively focuses on those falling below the poverty line and reflects the distribution of income (and dimensions of other wellbeing). However, average national income, which is a measure of central tendency, takes account of everyone’s income, and the super-rich can move the mean upward. In my previous study, I found out that aid per capita per country is explained by GNI per capita and population, but poverty rate does not have any significant explanatory power, or even if it does, it is in the negative direction (the poorer, the less aid). The ways economic growth and national income translate into poverty reduction differs by country; both income and poverty should be taken together. For instance, among countries with a similar average income per capita, should not those with a large proportion of poor be receiving more aid?
I also think that development communities should take advantage of the advancement of technology to measure poverty. We can validate and test the performance of new poverty measures through supervised learning, triangulate alternative measures, and use them to impute missing data. I found that the areas with the highest needs often have the least certain data, spatially and timely irrelevant. When serving these areas, even if the development community uses their best intentions, it is left with ad-hoc decisions to pick beneficiary communities. When the World Bank and Korea International Cooperation Agency started their community-centered development (CCD) projects in Myanmar in 2012-2013, the country didn’t have any reliable income and consumption data to identify the most impoverished townships or villages. The country’s first DHS data became available in 2015 and 2016, but proxy poverty measures such as the wealth index[3] are available in only 441 village clusters. Using geospatial interpolation techniques or poverty prediction techniques using satellite imagery, development communities can better pinpoint where the poor are and fill the development gaps using global social welfare program—development aid.
Your study concludes with a call for social work research and practice to return to the basics, and to begin by considering client needs. Why are you compelled to make this call?
Actually, I am speaking to the broad field of social science, including social welfare/social work and development engineering. I was compelled to make this call because a particular way of generating evidence may have obscured broader lessons. The knowledge continuum of a development project is composed of need assessment, implementation, evaluation, and policy uptake. Each piece of evidence can contribute to creating a holistic sense of impact. There will be a cost involved in putting too much emphasis on one of the continuums (e.g., outcome evaluation), a specific sector (e.g., health), or scope (micro approach). For instance, rigorous experimental studies can tease out socio-economic impacts of interventions but are less likely to recover quantities that are useful for policy.
Similarly, too much emphasis on outcomes can result in disproportionate aid allocation to sectors with easy-to-measure outcomes, such as health, HIV/AIDS prevention, while stifling innovations with hard-to-reach populations. With the promise of the big data revolution, questions also arise over the value added—other than confirming what’s already been known—in the international development context. Many development projects have failed because they did not simply pass the scrutiny of the very first test: Does the intervention take precedence over all competing resources for individuals and communities in extreme deprivation? Is providing a laptop for a child really a priority for children suffering from lack of water or food and in a village without electricity?
The sub-field of social welfare/social work is heavily leaning towards health science while the sub-field dedicated to anti-poverty policies has been losing its ground, particularly in the U.S. Still, I am not quite convinced why studies covering individual health outcomes such as patients experiencing depression or sleeplessness are more likely to be funded than inquiries about poverty, inequality, or structural impediments to finding decent work, which might affect billions of people and many other social problems. Part of the reason would be the substantial funding streams exclusively earmarked to the health sector with concrete indicators for success. Science that advances health is important to both the rich and the poor, but science that reduces poverty would be only an issue for the poor. I think such an imbalance in social welfare and in social science as a whole can be partly remedied by going back to basics, starting from client and user needs.
Tell us about your effort to combine fine-grained spatial techniques with satellite imagery to assess aid allocation in data-sparse communities in Myanmar. What did that involve, and what did you discover?
My efforts focused on creating poverty variables, combining spatial analysis and remote sensing methods. They involve the entire process of data science techniques—atomized data collection, the representation of non-traditional data, downstream machine learning tasks, and data visualization. Like in many other countries, Myanmar does not have poverty data at a small community level where aid projects are taking place. This would make it difficult to say whether aid-receiving communities are poorer than non-aid receiving communities or whether aid volume is explained by the degree of wealth. I used spatial interpolation techniques to overlay the gridded wealth field onto the georeferenced aid project locations, so that we can estimate the level of poverty in project villages as compared to non-project villages. The fine-grained spatial analysis also allows measurement of poverty at a small scale such as a 5 km by 5 km square grid depending on the resolution of the images, and it does not depend on administrative boundaries. What I also found interesting is that there are multiple ways of measuring poverty or needs broadly so that we can link needs and interventions. One of those is a distance to conflict areas from project villages, a measure of need relevant to fragile and conflict-prone countries. Beyond spatial interpolation, I also use nontraditional data sources such as daytime and nighttime satellite images. For instance, annual average nighttime luminosity across Myanmar was extracted from raster/image files and was trained to predict poverty using a convolutional neural network.
Through this new approach, I discovered mixed evidence in needs-based targeting. Community centered development (CCD) in Myanmar disproportionately flows to better-off communities, as indicated by a lower share of vulnerable populations per township and areas that shine brighter. However, unlike the literature that argues that aid favors the richest, my study suggests that a need-based allocation is also in place in Myanmar, at least for community-centered development, an aid instrument known for its emphasis on participation and inclusion. The previous studies used aggregated poverty measures at the state level, which is the highest administrative level, across African countries. Within villages of similar levels of population and electrification, aid goes to areas with low assets. The analytic tool I developed also helped me answer other questions. I found that the donor’s ideology shapes the design of aid projects design and project design matters in targeting. One CCD project concentrates on poorer regions, while the other project supports villages close to conflict zones.
Why did you choose to get a designated emphasis in development engineering? What did the field bring to your dissertation and how might it shape your academic career?
With a policy analyst background in development agencies, I wanted to continue work on international development and was about to start a concurrent MA in economics while earning a PhD. At that time, I also discovered the development engineering program and sought advice from Dr. Clair Brown to weigh in. I like what the program is aiming for—that is, addressing poverty by emphasizing human-centered design, adapting technology to local needs, and scaling up interventions. So I decided to take a route to development engineering.
I took core development engineering courses and was connected with innovative projects and their research teams, such as the Darfur Cook Stove project. That inspired me a lot, so for the last chapter of my dissertation, I wanted to survey “technology-informed data-intensive projects” (e.g., Development Impact Lab projects supported by the Blum Center) and interview principal investigators. However, after the discussion with the Blum Center, I realized that there is no centralized reservoir/data warehouse to collect such data. Due to this obstacle in doing a study of other studies, I thought, “Why don’t I get involved in data-savvy research?” and I ended up doing such research. The rigorous core and elective course of development engineering paved my way toward building data fluency and programming skills.
As I acknowledged in my dissertation, being part of the development engineering group has expanded my area of interest to the application of technology for social good. I really benefited from the marriage between STEM and social science education. For instance, I drew my aid occurrence and density outcome variable from spatial differences in African elephant densities. The development engineering program helped me select rigorous data science and impact evaluation courses to promote my analytic skills. It put me in touch with faculty members from various disciplines. The guidance and mentorship from my advisor, Dr. Brown, as well as Dr. Agogino and Dr. Levin, have been strong. Dr. Brown has been nourishing my scholarship in every way from the formulation of the research question to coaching for a job interview, to following up with article submission. The NSF INFEWS fellowship was also a tremendous financial support to pursue my dissertation.
The data science training and my interdisciplinary background with social welfare, public policy, and development dngineering will profoundly shape my academic career. I believe my unique contribution to the field is showing how to harness technology and data to identify the needs of the most impoverished in the world—from the eyes of social work, as well as for its direct work experience with clients.
—Tamara Straus
[1] Particularly low policy score (CPIA) countries receive more assistance to the civil service and governance subsector, which was a sub-sector that led to the increase in aid to the social sector.
[2] For instance, Zimbabwe in 2016 received a higher ratio of social sector aid (USD 151) despite its income poverty status. In contrast, Sudan in 2010 received a lower rate of social sector aid (USD 6.77) despite its capability poor status.
[3] Although the wealth index cannot be used directly to construct benchmark measures of poverty, these asset-based measures are capable of capturing a household’s long-term economic welfare in poor regions lacking consumption, expenditure and price data.